APPLYING BAYESIAN NETWORKS IN STUDENT DROPOUT DATA
Abstract:
This paper presents a proposal to implement a cluster method that best engages the educational data (socio-economic, academic achievement and dropouts) at the Engineering Faculty of Quevedo State Technical University. The use of graphical probabilistic models in the field of education has been proposed for this research. To complete the student diagnosis, and to pbkp_redict their behavior as well, an analysis of such Bayesian networks learning models, as PC, K2, and EM optimization was made first. There should be a test for each case where the probability is measured in every model using propagation algorithms. Then, probability logarithm is applied to each case and the results are added in each model to determine the best fit for the proposed. The results of this research will help raise awareness of the various factors affecting students’ performance. Besides, this will allow institutional authorities to identify mechanisms for improving retention index and students’ academic achievement, what serves the improvement of quality indicators in face of institutional and program evaluation and accbkp_reditation processes.
Año de publicación:
2022
Keywords:
- K2
- EM
- ACADEMIC PERFORMANCE
- Pc
- Bayesian networks
Fuente:
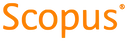
Tipo de documento:
Article
Estado:
Acceso restringido
Áreas de conocimiento:
- Aprendizaje automático
Áreas temáticas:
- Ciencias de la computación