Cubism: Co-balanced Mixup for Unsupervised Volcano-Seismic Knowledge Transfer
Abstract:
Volcanic eruptions are severe global threats. Forecasting these unrests via monitoring precursory earthquakes is vital for managing the consequent economic and social risks. Due to various contextual factors, volcano-seismic patterns are not spatiotemporal invariant. Training a robust model for any novel volcano-seismic situation relies on a costly, time-consuming and subjective process of manually labeling data; using a model trained on data from another volcano-seismic setting is typically not a viable option. Unsupervised domain adaptation (UDA) techniques address this issue by transferring knowledge extracted from a labeled domain to an unlabeled one. A challenging problem is the inherent imbalance in volcano-seismic data that degrades the efficiency of an adopted UDA technique. Here, we propose a co-balanced UDA approach, called Cubism, to bypass the manual annotation process for any newly monitored volcano by utilizing the patterns recognized in a different volcano-seismic dataset with labels. Employing an invertible latent space, Cubism alternates between a co-balanced generation of semantically meaningful inter-volcano samples and UDA. Inter-volcano samples are generated via the mixup data augmentation technique. Due to the sensitivity of mixup to data imbalance, Cubism introduces a novel co-balanced ratio that regulates the generation of inter-volcano samples considering the conditional distributions of both volcanoes. To the best of our knowledge, Cubism is the first UDA-based approach that transfers volcano-seismic knowledge without any supervision of an unseen volcano-seismic situation. Our extensive experiments show that Cubism significantly outperforms baseline methods and effectively provides a robust cross-volcano classifier.
Año de publicación:
2023
Keywords:
- Flow-based generative models
- Mixup
- Unsupervised domain adaptation
- Volcano-seismic event classification
- Imbalanced data
Fuente:
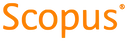
Tipo de documento:
Conference Object
Estado:
Acceso restringido
Áreas de conocimiento:
- Volcanismo
- Aprendizaje automático
Áreas temáticas:
- Ciencias de la computación