Current and emerging trends in medical image segmentation with deep learning
Abstract:
In recent years, the segmentation of anatomical or pathological structures using deep learning has experienced a widespread interest in medical image analysis. Remarkably successful performance has been reported in many imaging modalities and for a variety of clinical contexts to support clinicians in computer-assisted diagnosis, therapy or surgical planning purposes. However, despite the increasing amount of medical image segmentation challenges, there remains little consensus on which methodology perform best. Therefore, we examine in this paper the numerous developments and breakthroughs brought since the rise of U-Net inspired architectures. Especially, we focus on the technical challenges and emerging trends that the community is now focusing on, including conditional generative adversarial and cascaded networks, medical Transformers, contrastive learning, knowledge distillation, active learning, prior knowledge embedding, cross-modality learning, multi-structure analysis, federated learning or semi-supervised and self-supervised paradigms. We also suggest possible avenues to be further investigated in future research efforts.
Año de publicación:
2023
Keywords:
- market research
- Medical Imaging
- shape
- Medical diagnostic imaging
- image segmentation
- Deep Neural Networks
- Task analysis
- Semantic Segmentation
- TRANSFORMERS
- Artificial Intelligence
- Computer Architecture
- vision Transformers
Fuente:
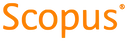
Tipo de documento:
Article
Estado:
Acceso restringido
Áreas de conocimiento:
- Aprendizaje automático
- Ciencias de la computación
Áreas temáticas de Dewey:
- Farmacología y terapéutica
- Enfermedades
- Ciencias de la computación