Cycle generative adversarial network: Towards a low-cost vegetation index estimation
Abstract:
This paper presents a novel unsupervised approach to estimate the Normalized Difference Vegetation Index (NDVI). The NDVI is obtained as the ratio between information from the visible and near infrared spectral bands; in the current work, the NDVI is estimated just from an image of the visible spectrum through a Cyclic Generative Adversarial Network (CyclicGAN). This unsupervised architecture learns to estimate the NDVI index by means of an image translation between the red channel of a given RGB image and the NDVI unpaired index’s image. The translation is obtained by means of a ResNET architecture and a multiple loss function. Experimental results obtained with this unsupervised scheme show the validity of the implemented model. Additionally, comparisons with the state of the art approaches are provided showing improvements with the proposed approach.
Año de publicación:
2021
Keywords:
Fuente:
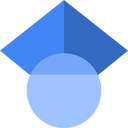
Tipo de documento:
Other
Estado:
Acceso abierto
Áreas de conocimiento:
- Aprendizaje automático
- Ciencias de la computación
- Sensores remotos
Áreas temáticas:
- Métodos informáticos especiales