DMS/SCADA data filtering using neural network tool to mid-term load forecasting
Abstract:
This paper presents a methodology for mid-term load forecasting using Artificial Neural Networks (ANN). The inputs to ANN are real time data available from Supervisory Control and Data Acquisition and Distribution Management Systems (SCADA/DMS) databases. Due to a number of reasons, historical data stored in SCADA/DMS databases is affected by distorted measurements that can jeopardize the load forecasting results. This paper explores mid-term load demand forecasting using ANN considering distorted measurements in SCADA/DMS database. Proposed technique was applied to real-world measurements acquired from a 8.3 kV substation in Venezuela. ANN's forecasted results are compared with an exponential smoothing load forecasting procedure.
Año de publicación:
2014
Keywords:
Fuente:
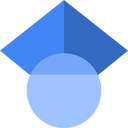
Tipo de documento:
Other
Estado:
Acceso abierto
Áreas de conocimiento:
- Aprendizaje automático
- Algoritmo
Áreas temáticas:
- Métodos informáticos especiales