DOMAIN ADAPTATION IN IMAGE DEHAZING: EXPLORING THE USAGE OF IMAGES FROM VIRTUAL SCENARIOS
Abstract:
This work presents a novel domain adaptation strategy for deep learning-based approaches to solve the image haze removal problem. Firstly, a large set of synthetic images is generated by using a realistic 3D graphic simulator; these synthetic images contain different densities of haze, which are used for training the model that is later adapted to any real scenario. The adaptation process requires just a few images to fine-tune the model parameters. The proposed strategy allows overcoming the limitation of training a given model with few images. In other words, the proposed strategy implements the adaptation of a haze removal model trained with synthetic images to real scenarios. It should be noticed that it is quite difficult, if not impossible, to have large sets of pairs of real-world images (with and without haze) to train in a supervised way haze removal algorithms. Experimental results are provided showing the validity of the proposed domain adaptation strategy.
Año de publicación:
2022
Keywords:
- Synthetic Hazed Dataset
- Domain Adaptation
- Dehazing
Fuente:
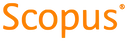
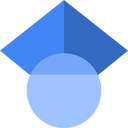
Tipo de documento:
Conference Object
Estado:
Acceso restringido
Áreas de conocimiento:
- Visión por computadora
- Ciencias de la computación
Áreas temáticas de Dewey:
- Métodos informáticos especiales