Damage Detection in an Offshore Experimental Wind Turbine Foundation based on Autoencoder Deep Neural Networks
Abstract:
The rapid growth of the energy capacity generated by wind turbines (WT), as well as their size, creates new challenges in terms of operation and maintenance. Structural health monitoring (SHM) strategies allow real-time structural condition diagnosis, which helps to detect damage earlier and thus leads to the adoption of efficient maintenance and repair measures. Offshore WTs are based on the same principle as traditional onshore models. However, their main difference lies in the extreme environmental conditions in which they operate due to higher wind speeds, and the effects of waves and currents. This work proposes a SHM methodology for structural damage detection in jacket-type offshore foundations for WTs. The proposed method analyzes the vibrational response of the sensor signals, based on the principle that any damage or modification to the structure will produce a change in its dynamic response. The WT has been considered to be subject to a crack damage located at different levels of the structure. The damage detection method presented in this work is an unsupervised deep learning algorithm based on an autoencoder deep neural network (ADNN) and its reconstruction error. One of the challenges of this research is that the input excitation signal (the wind) is unknown. The proposed approach is experimentally validated in a laboratory small-scale structure and the obtained results manifest the reliability of the stated fault diagnosis method.
Año de publicación:
2021
Keywords:
- ADNN
- wind turbines
- vibrational response
- dynamic response
- SHM
- damage detection
Fuente:
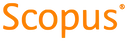
Tipo de documento:
Conference Object
Estado:
Acceso restringido
Áreas de conocimiento:
- Energía renovable
- Aprendizaje automático
Áreas temáticas de Dewey:
- Física aplicada
- Métodos informáticos especiales