Data Augmentation for Radio Frequency Fingerprinting via Pseudo-Random Integration
Abstract:
Radio frequency fingerprinting (RFF) is a lightweight access authentication method for the mobile terminal nodes. The classification accuracy of RFF is effectively improved by machine learning, of which the performance is deeply enhanced with the increasing training data. Data augmentation is the most frequently used method to get additional data and superior results with limited data in the classification of images and sounds. This paper proposes a random integration based data augmentation for signal processing via exploring the similarity between signal and deoxyribonucleic acid sequence. An optimized data augmentation based on pseudo-random integration is designed in order to provide additional data, and reduce the instability of classification accuracy caused by randomness simultaneously. Experiments are made to demonstrate performance of each augmentation method using the same model that classifies ten kinds of RF fingerprints with Gaussian support vector machine classifier, and show that pseudo-random integration has an obviously accurate and stable result with the same initial dataset and system, which implies that the performance of RFF could be improved further by applying pseudo-random integration based data augmentation.
Año de publicación:
2020
Keywords:
- random integration
- data augmentation
- radio frequency fingerprinting
- signal classification
- SVM
Fuente:
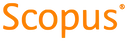