Data analysis using Riemannian geometry and applications to chemical engineering
Abstract:
We explore the use of tools from Riemannian geometry for the analysis of symmetric positive definite matrices (SPD). An SPD matrix is a versatile data representation that is commonly used in chemical engineering (e.g., covariance/correlation/Hessian matrices and images) and powerful techniques are available for its analysis (e.g., principal component analysis). A key observation that motivates this work is that SPD matrices live on a Riemannian manifold and that implementing techniques that exploit this basic property can yield significant benefits in data-centric tasks such as classification and dimensionality reduction. We demonstrate this via a couple of case studies that conduct anomaly detection in the context of process monitoring and image analysis.
Año de publicación:
2022
Keywords:
Fuente:
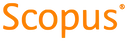
Tipo de documento:
Article
Estado:
Acceso restringido
Áreas de conocimiento:
- Ingeniería química
- Modelo matemático
Áreas temáticas:
- Análisis