Data mining process for identification of non-spontaneous saccadic movements in clinical electrooculography
Abstract:
In this paper we evaluate the use of the machine learning algorithms Support Vector Machines (SVM), K-Nearest Neighbors (KNN) and Classification and Regression Trees (CART) to identify non-spontaneous saccades in clinical electrooculography tests. We propose a modification to an adaptive threshold estimation algorithm for detecting signal impulses without the need for any manually pre-established parameters. Data mining tasks such as feature selection and model tuning were performed, obtaining very efficient models using only 3 attributes: amplitude deviation, absolute response latency and relative latency. The models were evaluated with signals recorded from subjects affected by Spinocerebellar Ataxia type 2 (SCA2). Results obtained by the algorithm show accuracies over 98%, recalls over 98% and precisions over 95% for the three models evaluated.
Año de publicación:
2017
Keywords:
- Classification and regression trees
- Saccade identification
- SUPPORT VECTOR MACHINES
- K-NEAREST NEIGHBORS
- Clinical electrooculography
Fuente:
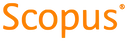
Tipo de documento:
Article
Estado:
Acceso restringido
Áreas de conocimiento:
- Minería de datos
Áreas temáticas de Dewey:
- El libro

Objetivos de Desarrollo Sostenible:
