Data-Driven Gearbox Fault Severity Diagnosis Based on Concept Drift
Abstract:
Condition-based maintenance aims to determine the machine state in real time, by monitoring the signals it emits. Such signals are potentially unlimited, generated at a high rate, and can evolve over time. These conditions tend to produce changes in the distribution of the data, known as concept drift. This phenomenon is analyzed and used to establish changes in the state of the machine. The present article proposes a methodological framework for the diagnosis of fault severity based on concept drift. A parsimonious unsupervised algorithm based on KNN is proposed to detect concept evolution. The results show that the algorithm is quite effective in declaring a concept evolution that is associated with a change in the failure condition of the machine. Finally, the results show that there is a high correlation between the displacement of the centroids of the emerging concepts and the % of deterioration of the machine.
Año de publicación:
2021
Keywords:
- Kullback-Leibler divergence
- concept evolution
- Fault Detection
- Concept drift
- Jensen-Shannon divergence
Fuente:
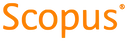
Tipo de documento:
Conference Object
Estado:
Acceso restringido
Áreas de conocimiento:
- Ciencias de la computación
- Aprendizaje automático
Áreas temáticas:
- Ciencias de la computación