Data-Driven Solutions for Electricity Price Forecasting: The Case of EU Improvement Project
Abstract:
Electricity is a necessity in all areas, which is why electricity consumption is increasing. With the renewable energy revolution, the variability of electricity prices has also increased. Knowing the price of electricity becomes essential to balance electricity production and consumption. In particular, for energy management systems (EMS) integrated into smart grids, long short-term electricity price forecasting is an endeavor to help control battery operators and energy demand response aggregators. In this study, we use both ordinary machine learning regression algorithms, namely Random Forest, Support vector machine, and XGBoost regressors, and a long short-term memory-based deep learning model (LSTM), which is known to solve nonlinear regression and time series problems. In several studies on electricity price pbkp_rediction, LSTM has been used to solve a univariate time series problem, in this study, we use LSTM for a multivariate time series problem. Where the data on the hourly aggregated renewable and non-renewable energies, is used to pbkp_redict the electricity prices. The results show that LSTM gives outstanding pbkp_redictions compared to machine learning algorithms where time is not considered an important factor for electricity price pbkp_rediction.
Año de publicación:
2022
Keywords:
- LSTM multivariate
- Electricity price pbkp_rediction
- long short-Term memory
Fuente:
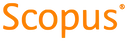
Tipo de documento:
Conference Object
Estado:
Acceso restringido
Áreas de conocimiento:
- Política energética
- Análisis de datos
- Energía
Áreas temáticas:
- Programación informática, programas, datos, seguridad
- Economía
- Física aplicada