Data-driven probabilistic power flow analysis for a distribution system with renewable energy sources using Monte Carlo simulation
Abstract:
This paper investigates the effect of uncertainty in the allocation of photovoltaic (PV) generation, solar irradiance, and its impact on the power flow in a distribution network. The solar irradiance available in the National Renewable Energy Laboratory Resource Data Center is clustered into two states: High and low irradiance defined by a threshold. The uncertainty is modeled based on Non-Gaussian distribution, obtained using kernel density estimation. This estimation aids in achieving the probability density function and cumulative distribution functions of the solar irradiance. Moreover, the load demand, wind speed, and generator location are modeled according to Gaussian, Weibull, and discrete uniform distribution functions, respectively. As a part of probabilistic power flow, the backward/forward sweep method is used to solve each scenario of the Monte Carlo simulation. The proposed framework is applied to the 33-node test system considering three different test cases. The first case considers deployment of PV systems in three microgrids of the electric grid, and the other two test cases analyze different levels of penetration of randomly allocated PV and wind power systems. At the end, the results indicate potential reverse power flow through certain branches of the grid, and the renewables have a major impact on the system.
Año de publicación:
2019
Keywords:
- photovoltaic (PV) systems
- renewable energy sources
- Monte Carlo simulation (MCS)
- Distributed Energy Resources (DERs)
- Kernel Density Estimation
- proabilistic power flow
Fuente:
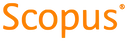
Tipo de documento:
Conference Object
Estado:
Acceso restringido
Áreas de conocimiento:
- Energía
- Energía
Áreas temáticas:
- Ciencias de la computación
- Física aplicada
- Métodos informáticos especiales