Data-driven techniques for modelling the gross primary production of the páramo vegetation using climate data: Application in the Ecuadorian Andean region
Abstract:
As one of the main areas of carbon cycle and climate change studies, water and CO2 relations are of great significance for estimation of gross primary production (GPP). Various biogeochemical process-based models have been set up to estimate the GPP based on mathematical representation of biological, physiological and ecological processes. However, they ended up increasing the complexity and computational processing power due to the large number of physical equations that need to be solved. Computational time becomes an important matter in the simulation of multiple scenarios using models for long periods of time (e.g. climate projections). Data driven surrogate models have proven to be a useful tool for environmental modelling especially when ecological and climatic co-variates are large. The advantages of Data Driven Models (DDM) are: the possibility of adding new independent variables even if their understanding is weak, and short computational time to run. The aim is to explore the ability of DDMs to replicate a biochemical model calculating GPP. This study evaluates the performance of four surrogate DDMs, namely linear regression method (LRM), model tree (MT), instance-based learning (IBL) and artificial neural network (ANN). A simple empirical and semi-empirical relationship between GPP and climatic variables are studied. Input variable selection (IVS) methods were used to decide on the most relevant and potential environmental model inputs and then followed by a two-step approach which included a model-free and a model-based technique. Data from the highlands (páramo ecosystem) in the Ecuadorian Andean Region from 12-year time-series (2000 − 2011) were used to evaluate the models at various time frames and at different altitudes. The GPP time series data for the same period were derived from an earlier study using the biomodel BIOME-BGC (BioGeochemical Cycles), which is a comprehensive physical based model used in different analysis of carbon fluxes around the world. So-called IBL (nearest neighbour method) showed a great capability to reproduce the GPP when data was aggregated to monthly time frame. The computational time used to evaluate the time series with IBL as the selected DDM is shorter with enough accuracy for using it in multi-model runs.
Año de publicación:
2018
Keywords:
- Vegetation models
- k-nearest neighbour
- neural network
- Biome-BGC
- Surrogate model
- Model tree
Fuente:
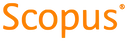
Tipo de documento:
Article
Estado:
Acceso restringido
Áreas de conocimiento:
- Ecología
- Ecología
- Ecología
Áreas temáticas:
- Agricultura y tecnologías afines
- Física aplicada
- Ecología