Decomposition-Based Multi-objective Landscape Features and Automated Algorithm Selection
Abstract:
Landscape analysis is of fundamental interest for improving our understanding on the behavior of evolutionary search, and for developing general-purpose automated solvers based on techniques from statistics and machine learning. In this paper, we push a step towards the development of a landscape-aware approach by proposing a set of landscape features for multi-objective combinatorial optimization, by decomposing the original multi-objective problem into a set of single-objective sub-problems. Based on a comprehensive set of bi-objective and three variants of the state-of-the-art Moea/d algorithm, we study the association between the proposed features, the global properties of the considered landscapes, and algorithm performance. We also show that decomposition-based features can be integrated into an automated approach for predicting algorithm performance and selecting the most accurate one on blind instances. In particular, our study reveals that such a landscape-aware approach is substantially better than the single best solver computed over the three considered Moea/d variants.
Año de publicación:
2021
Keywords:
Fuente:
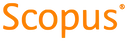
Tipo de documento:
Conference Object
Estado:
Acceso restringido
Áreas de conocimiento:
- Algoritmo
- Algoritmo
- Algoritmo
Áreas temáticas de Dewey:
- Ciencias de la computación

Objetivos de Desarrollo Sostenible:
