Deep Learning Architecture for Group Activity Recognition using Description of Local Motions<sup>∗</sup>
Abstract:
Nowadays, the recognition of group activities is a significant problem, specially in video surveillance. It is increasingly important to have vision architectures that automatically allow timely recognition of group activities and pbkp_redictions about them in order to make decisions. This paper proposes a computer vision architecture able to learn and recognise group activities using the movements of it in the scene. It is based on the Activity Description Vector (ADV), a descriptor able to represent the trajectory information of an image sequence as a collection of the local movements that occur in specific regions of the scene. The proposal evolves this descriptor towards the generation of images able to be the input queue of a two-stream convolutional neural network capable of robustly classifying group activities. Hence, this proposal, besides the use of trajectory analysis that allows a simple high level understanding of complex groups activities, takes advantage of the deep learning characteristics providing a robust architecture for multi-class recognition. The architecture has been evaluated and compared to other approaches using BEHAVE and INRIA dataset sequences obtaining great performance in the recognition of group activities.
Año de publicación:
2020
Keywords:
- video surveillance
- deep learning
- group activity recognition
- convolutional neural networks
- D-ADV
Fuente:
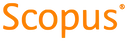
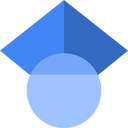
Tipo de documento:
Conference Object
Estado:
Acceso restringido
Áreas de conocimiento:
- Aprendizaje automático
- Ciencias de la computación
Áreas temáticas:
- Métodos informáticos especiales
- Ciencias de la computación
- Psicología aplicada