Deep Neural Nets for DOA Estimation with Random Arrays
Abstract:
An ensemble deep neural net framework is introduced to process nonuniform antenna arrays with unknown relative positions. The architecture is composed of two successive learning stages, the first one is a multilayered perceptron model and is used to interpolate the randomly sampled signals and estimate the corresponding uniformly sampled equivalent of the incoming signal. The second layer is an Long Short Term Memory network which learns the mapping of the incoming signal to its source location. The architecture allows for the model to learn the complex nonlinear functions required for mapping the arbitrarily sampled incoming space-time field to a virtual uniformly sampled field one and subsequently map it to the direction of the source. The structure has been trained and tested with independently generated sample sets, with results competitive with other algorithms that need the information about the sensor position.
Año de publicación:
2020
Keywords:
- Neural networks
- Array synthesis
- Direction of arrival estimation
- deep learning
- LSTM
- Adaptive arrays
Fuente:
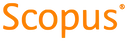
Tipo de documento:
Conference Object
Estado:
Acceso restringido
Áreas de conocimiento:
- Aprendizaje automático
Áreas temáticas:
- Ciencias de la computación