Deep ensemble-based classifier for transfer learning in rotating machinery fault diagnosis
Abstract:
Nowadays, intelligent models can correctly detect faults by analysing signals from rotating machinery. However, most of the studies are run in controlled environments and the performance in industrial real-world environments is not yet fully validated. Hence, a suitable tool to implement fault diagnosers is transfer learning, this topic is under development and challenges persist. This paper proposes a framework for creating accurate 1D-CNN based fault classifiers that can be transferred between different rotating machines and working conditions. Multiple Bayesian processes select architecture parameters and hyperparameters, which minimize a loss function related to their transferability to other machines and to the same machine under different operating conditions (such as load and engine speed). The resulting model is fitted to heterogeneous fault diagnosis data resulting in a 1D-CNN ensemble that improves …
Año de publicación:
2022
Keywords:
Fuente:
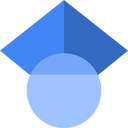
Tipo de documento:
Other
Estado:
Acceso abierto
Áreas de conocimiento:
- Aprendizaje automático
Áreas temáticas:
- Física aplicada
- Ciencias de la computación