Deep learning architecture for the recursive patterns recognition model
Abstract:
In this work, we propose a deep learning approach for the recursive pattern recognition model, called AR2P (for its acronym in Spanish: "Algoritmo Recursivo de Reconocimiento de Patrones"), by extending its supervised learning capability towards a semi-supervised learning scheme. The deep learning architecture is composed of three phases: The first one, called discovery phase, discovers the atomic descriptors. The second one, called aggregation phase, creates a feature hierarchy (merge of descriptors) from atomic descriptors. Finally, the classification phase carries out the classification of the inputs based on the feature hierarchy. The last phase uses a supervised learning approach, while the first two follow an unsupervised learning approach. In this paper is tested the performance of the proposed model, using a dataset from the UCI Machine Learning Repository.
Año de publicación:
2018
Keywords:
Fuente:
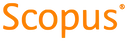
Tipo de documento:
Conference Object
Estado:
Acceso abierto
Áreas de conocimiento:
- Aprendizaje automático
- Algoritmo
- Ciencias de la computación
Áreas temáticas:
- Ciencias de la computación