Deep learning to implement a statistical weather forecast for the andean city of quito
Abstract:
A weather forecast is classically based on the use of a Physical Model computing information from Conventional Weather Stations (CWS), Automatic Weather Stations (AWS) and Geostationary Operational Environmental Satellite (GOES) imagery. For the Andean city of Quito, located at the latitude 0° this methodology turns to be inefficient and inaccurate. Several reasons can justify this problem but two can be highlighted: the sensibility of the Intertropical Convergence Zone and the geographic pattern of the Andean Region (microclimates). Both problems can be solved through the acquisition of new stations, nevertheless, this solution is expensive. In this paper, an investigative approach built on low cost small single-board computers (used as AWS) and Deep Learning is presented for solving the above. Eight neural networks for Temperature Forecast have been validated in base of the Walk Forward Technique. And temperature or Temperature/Humidity Time Series have been entered into the networks to find what could be the best inputs to generate the pbkp_redictions. Finally, errors between 0.74 °C and 2.24°C have been obtained from the pbkp_redictions and it was determined that the lowest values of error are obtained when one pbkp_rediction hinges on the temperature series of all the stations in the network.
Año de publicación:
2020
Keywords:
- deep learning
- Weather Forecast
- Neural networks
- automatic weather station
Fuente:
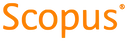
Tipo de documento:
Conference Object
Estado:
Acceso restringido
Áreas de conocimiento:
- Aprendizaje automático
- Meteorología
- Ciencias de la computación
Áreas temáticas:
- Ciencias de la computación
- Probabilidades y matemática aplicada
- Geología, hidrología, meteorología