Deep learning-based gear pitting severity assessment using acoustic emission, vibration and currents signals
Abstract:
A method for gearbox pitting faults severity classification using Deep Learning techniques is reported. The signals are preprocessed for obtaining a 2D time-frequency representation corresponding to the Mel Frequency Cepstral Coefficients. This bi-dimensional representation is the feature space used for classification. A Long Short Term Memory network (LSTM) is used for classifying nine levels of pitting in spur gears. Each signals dataset is used for training and validating a LSTM network. Classification accuracies up to 100 % are obtained during cross-validation with the analyzed signals dataset.
Año de publicación:
2019
Keywords:
Fuente:
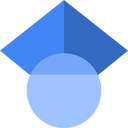
Tipo de documento:
Other
Estado:
Acceso abierto
Áreas de conocimiento:
- Ingeniería mecánica
- Aprendizaje automático
Áreas temáticas:
- Métodos informáticos especiales
- Física aplicada
- Ingeniería y operaciones afines