Demand Forecasting for Textile Products Using Machine Learning Methods
Abstract:
Due to its close relationship with various operational decisions, market demand forecasting has been considered one of the essential activities in all organizations. Unfortunately, the textile industry has been the most difficulty generating forecasts, mainly due to the volatility caused in the market by short product life cycles, special events, and competitions. From the beginning, forecasting has been using traditional statistical methods. However, the increasing use of artificial intelligence has opened a new catalog of prediction methods currently being studied for their high precision. This study explores machine learning (ML) as a tool to generate forecasts for the textile industry, applying regression-focused algorithms such as Linear Regression, Ridge, Lasso, K-nearest neighbor, Support Vector Regression (SVR), and Random Forest (RF). To this end, time series were used as inputs for the models, supported by external variables such as Google Trends and special events. The results show that ML as a prediction method has higher precision than purely statistical basic prediction models. Additionally, SVR and Ridge models obtain a lower error in metrics such as MSE (0.09787 and 0.09682), RMSE (0.31285 and 0.31117), and RSE (0.32977 and 0.32800), respectively. Meanwhile, Google Trends reduces the MSE error by 2% and 15%, RMSE 1% and 7% and, RSE 1% and 7% for SVR and Ridge models, respectively.
Año de publicación:
2022
Keywords:
- Demand Forecast
- TEXTILE INDUSTRY
- Machine learning
- google trends
Fuente:
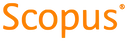
Tipo de documento:
Conference Object
Estado:
Acceso restringido
Áreas de conocimiento:
- Textil
- Aprendizaje automático
- Ciencias de la computación
Áreas temáticas de Dewey:
- Dirección general

Objetivos de Desarrollo Sostenible:
