DenseNet for Breast Tumor Classification in Mammographic Images
Abstract:
Breast cancer screening is an efficient method to detect breast lesions early. The common screening techniques are tomosynthesis and mammography images. However, the traditional manual diagnosis requires an intense workload for pathologists, and hence is prone to diagnostic errors. Thus, the aim of this study was to build a deep convolutional neural network method for automatic detection, segmentation, and classification of breast lesions in mammography images. Based on deep learning the Mask-CNN (RoIAlign) method was developed to automate RoI segmentation. Then feature extraction, selection and classification were carried out by the DenseNet architecture. Finally, the precision and accuracy of the model was evaluated by the AUC, accuracy and precision metrics. To summarize, the findings of this study show that the methodology may improve the diagnosis and efficiency in automatic tumor localization through medical image classification.
Año de publicación:
2021
Keywords:
- Breast tumor classification
- Mammography
- Convolutional neural network
- DenseNet
- RoI align
- deep learning
Fuente:
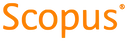
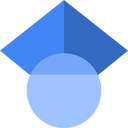
Tipo de documento:
Conference Object
Estado:
Acceso restringido
Áreas de conocimiento:
- Cáncer
- Ciencias de la computación
Áreas temáticas:
- Medicina y salud