Accelerating wireless channel autoencoders for short coherence-time communications
Abstract:
Traditional wireless communication theory is based on complex probabilistic models and fixed conjectures, which limit the optimal utilization of spectrum resources. Deep learning has been used to design end-to-end communication systems using an encoder to replace the transmitter and a decoder for the receiver. We address the challenge to update the parameters of a wireless channel autoencoder (AE) under a time-varying channel with short coherence-time. We suggest an optimized training algorithm that updates the learning rate value on a per-dimension basis, restricting the past gradients instead of accumulating them. We also scale the initial weights of our AE by sampling them from a normalized uniform distribution. While recently proposed AE configurations might fail to converge at a few number of epochs, our setting attains a fast convergence maintaining its robustness to large gradients, oscillations, and vanishing problems. By simulation results, we demonstrate that our proposed AE configuration improves the bit reconstruction accuracy in shorter training time.
Año de publicación:
2020
Keywords:
- Channel estimation
- physical layer
- Wireless systems
- Autoencoders
- deep learning
Fuente:
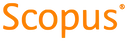
Tipo de documento:
Article
Estado:
Acceso abierto
Áreas de conocimiento:
- Comunicación
- Red inalámbrica
Áreas temáticas de Dewey:
- Ciencias de la computación