Accuracy' measures of sentiment analysis algorithms for spanish corpus generated in peer assessment
Abstract:
The purpose of this study is to test a model that classifies some sentiment as positive or negative from some feedback in Spanish that are generated through peer assessment in Higher Education. The Supervised Machine Learning method is implemented. Several experiments are performed with a manually tagged data set to test different combinations of N-grams with Term Frequency-Inverse Document Frequency (TF-IDF), and classification algorithms: Multinomial Naive Bayes, Support Vector Machine, Logistic Regression, and also Random Forest, in order to obtain the right combination that gives the best performance. The simulation results displayed that the Support Vector Machine classifier with the combination of 1-grams + 2-grams + TF-IDF is the best model in Precision, Recall and F-Measure.
Año de publicación:
2020
Keywords:
- higher education
- Peer Assessment
- Spanish corpus
- sentiment analysis
- classification algorithms
- Machine learning
Fuente:
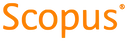
Tipo de documento:
Conference Object
Estado:
Acceso restringido
Áreas de conocimiento:
- Aprendizaje automático
- Idioma
Áreas temáticas:
- Programación informática, programas, datos, seguridad