Design and simulation of a path decision algorithm for a labyrinth robot using neural networks
Abstract:
This document consists of the design and simulation of a decision algorithm capable of solving labyrinths using neural networks under the sequential and LSTM recursive architec-tures.For this design, important elements are mentioned for the Q-learning algorithm and an Adam optimization algorithm through the MSE loss function. There are two types of charac-teristics: qualitative and quantitative, the first because Python need compatible libraries such as the following: Numpy, PyTorch, Matplotlib, Tkinter and Jupiter. The second is quantitative type for the construction of the Q-learning algorithm based on the states and actions that the agent performs to define the reward according to the short and long deadline objectives. Labyrinths are generated randomly so that the agent does not memorize the solution path for which tests were made and test the training of each neural network.
Año de publicación:
2022
Keywords:
- Q learning
- Deep reinforcement learning
- sequential neural network
- labyrinth
- recursive neural network
Fuente:
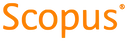
Tipo de documento:
Conference Object
Estado:
Acceso restringido
Áreas de conocimiento:
- Robótica
- Algoritmo
Áreas temáticas:
- Ciencias de la computación