Design of Hybrid Reconstruction Scheme for Compressible Flow Using Data-Driven Methods
Abstract:
Existing numerical schemes used to solve the governing equations for compressible flow suffer from dissipation errors which tend to smear out sharp discontinuities. Hybrid schemes show potential improvements in this challenging problem; however, the solution quality of a hybrid scheme heavily depends on the criterion to switch between the different candidate reconstruction functions. This work presents a new type of switching criterion (or selector) using machine learning techniques. The selector is trained with randomly generated samples of continuous and discontinuous data profiles, using the exact solution of the governing equation as a reference. Neural networks and random forests were used as the machine learning frameworks to train the selector, and it was later implemented as the indicator function in a hybrid scheme which includes THINC and WENO-Z as the candidate reconstruction functions. The trained selector has been verified to be effective as a reliable switching criterion in the hybrid scheme, which significantly improves the solution quality for both advection and Euler equations.
Año de publicación:
2020
Keywords:
- Hybrid Schemes
- Machine learning
- Compressible Flow
- Computational Fluid Dynamics
Fuente:
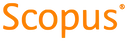
Tipo de documento:
Article
Estado:
Acceso restringido
Áreas de conocimiento:
- Aprendizaje automático
- Dinámica de fluidos
Áreas temáticas:
- Física aplicada
- Métodos informáticos especiales
- Ciencias de la computación