Design of Type-3 Fuzzy Systems and Ensemble Neural Networks for COVID-19 Time Series Pbkp_rediction Using a Firefly Algorithm
Abstract:
In this work, information on COVID-19 confirmed cases is utilized as a dataset to perform time series pbkp_redictions. We propose the design of ensemble neural networks (ENNs) and type-3 fuzzy inference systems (FISs) for pbkp_redicting COVID-19 data. The answers for each ENN module are combined using weights provided by the type-3 FIS, in which the ENN is also designed using the firefly algorithm (FA) optimization technique. The proposed method, called ENNT3FL-FA, is applied to the COVID-19 data for confirmed cases from 12 countries. The COVID-19 data have shown to be a complex time series due to unstable behavior in certain periods of time. For example, it is unknown when a new wave will exist and how it will affect each country due to the increase in cases due to many factors. The proposed method seeks mainly to find the number of modules of the ENN and the best possible parameters, such as lower scale and lower lag of the type-3 FIS. Each module of the ENN produces an individual pbkp_rediction. Each pbkp_rediction error is an input for the type-3 FIS; moreover, outputs provide a weight for each pbkp_rediction, and then the final pbkp_rediction can be calculated. The type-3 fuzzy weighted average (FWA) integration method is compared with the type-2 FWA to verify its ability to pbkp_redict future confirmed cases by using two data periods. The achieved results show how the proposed method allows better results for the real pbkp_rediction of 20 future days for most of the countries used in this study, especially when the number of data points increases. In countries such as Germany, India, Italy, Mexico, Poland, Spain, the United Kingdom, and the United …
Año de publicación:
2022
Keywords:
Fuente:
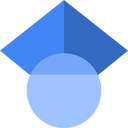
Tipo de documento:
Other
Estado:
Acceso abierto
Áreas de conocimiento:
- Aprendizaje automático
- Algoritmo
Áreas temáticas:
- Ciencias de la computación