Detecting Activation in fMRI Data: An Approach Based on Sparse Representation of BOLD Signal
Abstract:
This paper proposes a simple yet effective approach for detecting activated voxels in fMRI data by exploiting the inherent sparsity property of the BOLD signal in temporal and spatial domains. In the time domain, the approach combines the General Linear Model (GLM) with a Least Absolute Deviation (LAD) based regression method regularized by the pseudonorm l0 to promote sparsity in the parameter vector of the model. In the spatial domain, detection of activated regions is based on thresholding the spatial map of estimated parameters associated with a particular stimulus. The threshold is calculated by exploiting the sparseness of the BOLD signal in the spatial domain assuming a Laplacian distribution model. The proposed approach is validated using synthetic and real fMRI data. For synthetic data, results show that the proposed approach is able to detect most activated voxels without any false activation. For real data, the method is evaluated through comparison with the SPM software. Results indicate that this approach can effectively find activated regions that are similar to those found by SPM, but using a much simpler approach. This study may lead to the development of robust spatial approaches to further simplifying the complexity of classical schemes.
Año de publicación:
2018
Keywords:
Fuente:
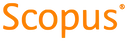
Tipo de documento:
Article
Estado:
Acceso abierto
Áreas de conocimiento:
- Aprendizaje automático
- Simulación por computadora
Áreas temáticas:
- Enfermedades
- Fisiología humana
- Sistemas fisiológicos específicos de los animales