Detecting Dependency between Discrete Random Variables and Application
Abstract:
Finding the relationship between two random variables is important because it brings insights to design appropriate probabilistic models for a given dataset. The problem with current measures like Maximal Information Coefficient (MIC) is that can take a considerable amount of time to compute it, thus faster methods are desirable. In this paper, we propose two novel measures capable to detect linear and nonlinear dependency between random variables. The proposed measures are used to design new correlation functions, in order to compare them with the ones in literature. These novel dependency measures have been applied to supervised feature selection to show their usefulness.
Año de publicación:
2019
Keywords:
- Machine learning
- random variable
- feature selection
- Correlation
- Dependency
Fuente:
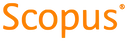
Tipo de documento:
Conference Object
Estado:
Acceso restringido
Áreas de conocimiento:
- Estadísticas
- Probabilidad
Áreas temáticas:
- Programación informática, programas, datos, seguridad