Detecting bearing failures in wind energy parks: A main bearing early damage detection method using SCADA data and a convolutional autoencoder
Abstract:
Wind energy maintenance and operation costs can total millions of dollars each year in an average industrial-size wind park. Therefore, moving from preventive and corrective maintenance to pbkp_redictive maintenance is imperative in the wind energy sector. This paper contributes to this challenge by providing a main bearing early damage detection technique that exclusively uses standard supervisory control and data acquisition (SCADA) data (10-min average) and a convolutional autoencoder with the following contributions. (i) Entirely semisupervised (not requiring the labeling of data through work order logs and avoiding the problem of data imbalance between classes) based only on healthy data, thus expanding its range of application (even when the failure of interest has never occurred in the park before). (ii) Validated using real-world SCADA data and shown to be resistant to seasonality, and operational and environmental conditions. (iii) Reliable pbkp_redictions with minimum false alarms thanks to specially designed fault prognosis indicators based on the image mean square error metric. (vi) The early warning is achieved months in advance, thus providing adequate time for plant operators to plan properly. (v) The main use of exogenous variables in the model (variables that are not affected by other variables, e.g., wind speed, wind turbulence, and ambient temperature) guarantees the detection of damage directly related only to the low-speed shaft temperature (the only nonexogenous variable used by the stated model). (vi) Finally, the proposed strategy is validated in a wind park made up of 12 wind turbines.
Año de publicación:
2023
Keywords:
- Wind turbine
- Fault Detection
- Autoencoder
Fuente:
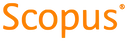
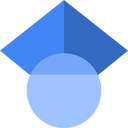
Tipo de documento:
Article
Estado:
Acceso abierto
Áreas de conocimiento:
- Energía renovable
- Aprendizaje automático
Áreas temáticas:
- Física aplicada
- Otras ramas de la ingeniería
- Ciencias de la computación