Detecting changes of transportation-mode by using classification data
Abstract:
Several techniques aim to classify human activity using data from sensors e.g., GPS, accelerometer, Wi-Fi and GSM. The sensor data allow inferring transportation modes as car, bus, walk, and bike. Despite some techniques show improvements in accuracy, researchers constantly deal with issues such as over-segmentation and low precision in trip reporting. Journeys are over-segmented due to the ambiguous situations, for instance: traffic lights, traffic jam, bus stops and weak signal reception. Thereby, current techniques report high misclassification errors. We present a method for detecting changes of transportation mode on a multimodal journey, where the input data regard to the classification of human activities. We use a space transformation for extracting features that identify a transition between two transportation modes. The data are collected from the Google API for Human Activity Classification through a crowdsourcing-based application for smartphones. Results show improvements on precision and accuracy in comparison to initial classification data outcomes. Therefore, our approach reduces the over-segmentation for multimodal journeys.
Año de publicación:
2015
Keywords:
- segmentation
- activity classification
- change mode detection
Fuente:
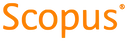
Tipo de documento:
Conference Object
Estado:
Acceso restringido
Áreas de conocimiento:
- Aprendizaje automático
- Transporte
Áreas temáticas:
- Otras ramas de la ingeniería
- Programación informática, programas, datos, seguridad
- Métodos informáticos especiales