Detecting convergence in genetic algorithms with decreasing mutation policies
Abstract:
The efficiency and effectiveness of Real-Coded Genetic Algorithms (RCGAs) are highly determined by the degree of exploitation and exploration kept throughout the run. In the cases that RCGAs use mutation operators whose rates decrease with the number of generations, having a metric of dissimilarity is very important. This metric may allow to evaluate the effectiveness of the crossover operator as the number of mutations at each iteration decreases, and it may provide a way to detect the convergence of the algorithm. The present work introduces a new method of detecting convergence of RCGAs that use decreasing mutation policies. This new procedure consists in the measuring of the diversity of the individuals of each generation by applying the modified Simpson's Diversity index, which is often used to quantify the biodiversity of a habitat in ecology.
Año de publicación:
2005
Keywords:
- convergence
- Optimization
- Diversity index
- Global optimization
- Genetic Algorithms
Fuente:
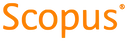
Tipo de documento:
Conference Object
Estado:
Acceso restringido
Áreas de conocimiento:
- Algoritmo
- Genética
- Algoritmo
Áreas temáticas:
- Conocimiento