Detection and identification of malicious cyber-attacks in connected and automated vehicles’ real-time sensors
Abstract:
Connected and automated vehicles (CAVs) as a part of Intelligent Transportation Systems (ITS) are projected to revolutionise the transportation industry, primarily by allowing real-time and seamless information exchange of vehicle-to-vehicle (V2V) and vehicle-to-infrastructure (V2I). However, these connectivity and automation are expected to offer vast numbers of benefits, new challenges in terms of safety, security and privacy also emerge. CAVs continue to rely heavily on their sensor readings, the input obtained from other vehicles and the road side units to inspect roadways. Consequently, anomalous reading of sensors triggered by malicious cyber attacks may lead to fatal consequences. Hence, like all other safety-critical applications, in CAVs also, reliable and secure information dissemination is of utmost importance. As a result, real time detection of anomaly along with identifying the source is a pre-requisite for mass deployment of CAVs. Motivated by this safety concerns in CAVs, we develop an efficient anomaly detection method through the combination of Bayesian deep learning (BDL) with discrete wavelet transform (DWT) to improve the safety and security in CAVs. In particular, DWT is used to smooth sensor reading of a CAV and then feed the data to a BDL module for analysis of the detection and identification of anomalous sensor behavior/data points caused by either malicious cyber attacks or faulty vehicle sensors. Our numerical experiments show that the proposed method demonstrates significant improvement in detection anomalies in terms of accuracy, sensitivity, precision, and F1-score evaluation metrics. For these metrics, the proposed method shows an average performance gain of 7.95%, 9% 8.77% and 7.33%, respectively when compared with Convolutional Neural Network (CNN-1D), and when compared with BDL, the corresponding numbers are 5%, 7.9% 7.54% and 4.1% respectively.
Año de publicación:
2020
Keywords:
- Bayesian deep learning
- Connected and automated vehicles
- Intelligent transportation system
- Discrete Wavelet Transform
- convolution neural network
Fuente:
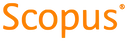
Tipo de documento:
Article
Estado:
Acceso abierto
Áreas de conocimiento:
- Red informática
- Ciencias de la computación
Áreas temáticas:
- Ciencias de la computación