Detection of desertion patterns in university students using data mining techniques: A case study
Abstract:
Student desertion is a phenomenon that affects higher education and academic quality standards. Several causes can lead to this issue, the academic factor being a potential reason. The main objective of this research is to detect dropout patterns in the “Técnica del Norte” University (Ecuador), based on personal and academic historical data, using pbkp_redictive classification techniques in data mining. The KDD (Knowledge Discovery in Databases) process was used to determine desertion patterns focused on two approaches: (i) Bayesian, and (ii) Decision Trees, both implemented on Weka. The classifiers performance was quantitatively evaluated using the confusion matrix and quality metrics. The results proved that the technique based on decision trees had slightly better performance than the Bayesian approach on the processed data.
Año de publicación:
2019
Keywords:
- Pattern discovery
- Student desertion
- Data Mining
- WEKA
- KDD
Fuente:
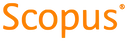
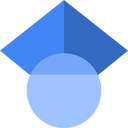
Tipo de documento:
Conference Object
Estado:
Acceso restringido
Áreas de conocimiento:
- Educación superior
- Minería de datos
Áreas temáticas:
- Conocimiento