Determination of climatic conditions related to precipitation anomalies in the Tropical Andes by means of the random forest algorithm and novel climate indices
Abstract:
Understanding precipitation and its relation with atmospheric and oceanic conditions is vital in the face of climate change. This is crucial in the Tropical Andes (TA) because millions of people depend on water originated in the cordillera. Unfortunately, the paucity of meteorological monitoring that exists in mountainous regions is accentuated in the tropics. In this context, climate indices, remotely sensed, and gridded datasets, are useful tools to study climate and precipitation in the TA, and additional climate indices can be calculated from reanalysis datasets. The combination of this information with traditional indices has the potential to improve our understanding of precipitation. Our objective was to use the k-means algorithm to regionalize precipitation in the TA (different regions have different climate), and then use the random forest algorithm to study the variables related to precipitation in each of these regions in seasonal timescales. Here, we show the suitability of the random forest algorithm to reveal climate processes and the high potential of the novel climate indices to improve the regressions. We found that convective available potential energy was the most important variable for precipitation in the northern TA, except for the Chocó, where v at 850 hPa was the most important one. Meanwhile, vertical integral of divergence of moisture flux was the most important one in the southern TA. Interestingly, in the DJF season when the South American low-level jet (SALLJ) is more active, u and v at 850 hPa showed their lowest relative importance and the total column of water vapour showed its maximum, this could indicate that precipitation anomalies are controlled by atmospheric moisture availability rather than by the speed of the SALLJ during DJF. These results deepen our understanding of precipitation anomalies in the TA and the related oceanic and atmospheric conditions. The proposed methodology was proven to be suitable and it could be used in the future to test and formulate new hypotheses, and to forecast seasonal precipitation.
Año de publicación:
2022
Keywords:
- tropics
- K-Means
- Machine learning
- rainfall
- Andes
- SOUTH AMERICA
- climate anomalies
- large-scale climate oscillations
Fuente:
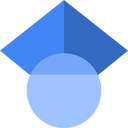
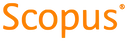
Tipo de documento:
Article
Estado:
Acceso restringido
Áreas de conocimiento:
- Clima
- Aprendizaje automático
- Clima
Áreas temáticas de Dewey:
- Geología, hidrología, meteorología

Objetivos de Desarrollo Sostenible:
- ODS 13: Acción por el clima
- ODS 6: Agua limpia y saneamiento
- ODS 9: Industria, innovación e infraestructura
