Development of Activity Rules and Chemical Fragment Design for In Silico Discovery of AChE and BACE1 Dual Inhibitors against Alzheimer’s Disease
Abstract:
Multi-target drug development has become an attractive strategy in the discovery of drugs to treat of Alzheimer’s disease (AzD). In this study, for the first time, a rule-based machine learning (ML) approach with classification trees (CT) was applied for the rational design of novel dual-target acetylcholinesterase (AChE) and β-site amyloid-protein precursor cleaving enzyme 1 (BACE1) inhibitors. Updated data from 3524 compounds with AChE and BACE1 measurements were curated from the ChEMBL database. The best global accuracies of training/external validation for AChE and BACE1 were 0.85/0.80 and 0.83/0.81, respectively. The rules were then applied to screen dual inhibitors from the original databases. Based on the best rules obtained from each classification tree, a set of potential AChE and BACE1 inhibitors were identified, and active fragments were extracted using Murcko-type decomposition analysis. More than 250 novel inhibitors were designed in silico based on active fragments and pbkp_redicted AChE and BACE1 inhibitory activity using consensus QSAR models and docking validations. The rule-based and ML approach applied in this study may be useful for the in silico design and screening of new AChE and BACE1 dual inhibitors against AzD.
Año de publicación:
2023
Keywords:
- AChE
- fragment design
- QSAR
- dual-target inhibitor
- BACE1
- Alzheimer’s disease
Fuente:
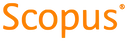
Tipo de documento:
Article
Estado:
Acceso abierto
Áreas de conocimiento:
- Descubrimiento de fármacos
Áreas temáticas:
- Funcionamiento de bibliotecas y archivos
- Problemas sociales y servicios a grupos
- Farmacología y terapéutica