Development of Machine Learning-based Pbkp_redictive Models for Air Quality Monitoring and Characterization
Abstract:
One of the biggest environmental problems right now is air pollution. Air quality is needed to be consistently monitored and assessed to ensure better living conditions. The U.S. Environmental Protection Agency (EPA) uses the air quality index (AQI) to standardize the air quality. However, AQI requires precise and accurate sensor readings and complex calculations, making it not feasible for portable air quality monitoring devices. The aim of this paper is to find an alternative way of monitoring and characterizing air quality through the use of integrated gas sensors and building pbkp_redictive models using machine learning algorithms that can be used to obtain data-driven solutions to mitigate the risk of air pollution. The proposed methodology is implemented by building a prototype for the integrated sensors using DHT 11 temperature and relative humidity sensor, MQ2, MQ5 and MQ135 gas sensors. Five pbkp_redictive models are developed in the study, k-nearest neighbors (KNN), support vector machine (SVM), Naïve-Bayesian classifier, random forest and neural network. Results show that the researchers are able to obtain an accuracy of 98.67%, 97.78%, 98.67%, 94.22%, and 99.56% for all the five models respectively, having the neural network to be the best performing model.
Año de publicación:
2019
Keywords:
- Integrated Sensors
- Machine learning
- r programming
- air quality characterization
- AQI
Fuente:
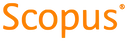
Tipo de documento:
Conference Object
Estado:
Acceso restringido
Áreas de conocimiento:
- Aprendizaje automático
- Contaminación del aire
- Ciencia ambiental
Áreas temáticas:
- Ciencias de la computación
- Otras ramas de la ingeniería
- Ingeniería sanitaria