Development of discharge-stage curves affected by hysteresis using time varying models, model trees and neural networks
Abstract:
Flow data forms the base on which much of the edifice of water management is raised. However, flow measurements are expensive and difficult to conduct. Therefore, the more accessible stage measurements are employed in combination with stage-discharge relationships. Setting up such relationships is often infeasible using traditional regression techniques. Two case studies are examined that show hystereses using various approaches, namely (1) single rating curves, (2) rating curves with dynamic correction, (3) artificial neural networks (ANN) and (4) M5' model trees. All methods outperform the traditional rating curve. The presented approach that uses a dynamically corrected rating curve delivers accurate results and allows for physical interpretation. The ANNs mimic the calibration data precisely, but suffer from overfitting when a small amount of data is applied for training. The rarely used M5' model tree's architecture is easier to interpret than that of neural networks and delivers more accurate results. © 2014 Elsevier Ltd.
Año de publicación:
2014
Keywords:
- State dependent parameter analysis
- Rating curve
- neural network
- Hysteresis
- regression
- Model tree
Fuente:
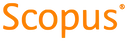
Tipo de documento:
Article
Estado:
Acceso restringido
Áreas de conocimiento:
- Optimización matemática
- Aprendizaje automático
- Hidrología
Áreas temáticas de Dewey:
- Ciencias de la computación

Objetivos de Desarrollo Sostenible:
- ODS 6: Agua limpia y saneamiento
- ODS 13: Acción por el clima
- ODS 9: Industria, innovación e infraestructura
