Developments on Support Vector Machines for Multiple-Expert Learning
Abstract:
In supervised learning scenarios, some applications require solve a classification problem wherein labels are not given as a single ground truth. Instead, the criteria of a set of experts is used to provide labels aimed at compensating for the erroneous influence with respect to a single labeler as well as the error bias (excellent or lousy) due to the level of perception and experience of each expert. This paper aims to briefly outline mathematical developments on support vector machines (SVM), and overview SVM-based approaches for multiple expert learning (MEL). Such MEL approaches are posed by modifying the formulation of a least-squares SVM, which enables to obtain a set of reliable, objective labels while penalizing the evaluation quality of each expert. Particularly, this work studies both two-class (binary) MEL classifier (BMLC) and its extension to multiclass through one-against all (OaA-MLC) including penalization of each expert’s influence. Formal mathematical developments are stated, as well as remarkable discussion on key aspects about the least-squares SVM formulation and penalty factors are provided.
Año de publicación:
2021
Keywords:
- Multiple expert learning
- SUPPORT VECTOR MACHINES
- Supervised learning
Fuente:
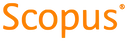
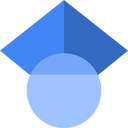
Tipo de documento:
Conference Object
Estado:
Acceso restringido
Áreas de conocimiento:
- Aprendizaje automático
- Ciencias de la computación
Áreas temáticas:
- Métodos informáticos especiales
- Ciencias de la computación
- Funcionamiento de bibliotecas y archivos