Different perspectives for kernel spectral clustering: A theoretical study
Abstract:
Spectral clustering is a suitable technique to deal with problems involving unlabeled clusters and having a complex structure, being kernel-based approaches the most recommended ones. This work aims at demonstrating the relationship between a widely-recommended method, so-named kernel spectral clustering (KSC) and other well-known approaches, namely normalized cut clustering and kernel k-means. Such demonstrations are done by following a primal-dual scheme. Also, we mathematically and experimentally prove the usability of using LS-SVM formulations with a model. Experiments are conducted to assess the clustering performance of KSC and the other considered methods on image segmentation tasks.
Año de publicación:
2016
Keywords:
- SUPPORT VECTOR MACHINES
- Kernel
- Spectral clustering
Fuente:
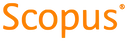
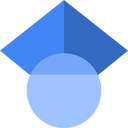
Tipo de documento:
Conference Object
Estado:
Acceso restringido
Áreas de conocimiento:
- Aprendizaje automático
- Optimización matemática
Áreas temáticas de Dewey:
- Ciencias de la computación

Objetivos de Desarrollo Sostenible:
- ODS 9: Industria, innovación e infraestructura
- ODS 17: Alianzas para lograr los objetivos
- ODS 4: Educación de calidad
