Dimensionality reduction based on fuzzy rough sets oriented to ischemia detection
Abstract:
This paper presents a dimensionality reduction study based on fuzzy rough sets with the aim of increasing the discriminant capability of the representation of normal ECG beats and those that contain ischemic events. A novel procedure is proposed to obtain the fuzzy equivalence classes based on entropy and neighborhood techniques and a modification of the Quick Reduct Algorithm is used to select the relevant features from a large feature space by a dependency function. The tests were carried out on a feature space made up by 840 wavelet features extracted from 900 ECG normal beats and 900 ECG beats with evidence of ischemia. Results of around 99% classification accuracy are obtained. This methodology provides a reduced feature space with low complexity and high representation capability. Additionally, the discriminant strength of entropy in terms of representing ischemic disorders from time-frequency information in ECG signals is highlighted. © 2012 IEEE.
Año de publicación:
2012
Keywords:
Fuente:
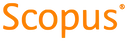
Tipo de documento:
Conference Object
Estado:
Acceso restringido
Áreas de conocimiento:
- Aprendizaje automático
- Laboratorio médico
Áreas temáticas:
- Ciencias de la computación
- Educación
- Medicina y salud