Adapting regression equations to minimize the mean squared error of pbkp_redictions made using covariate data from a gis
Abstract:
Regression equations between a response variable and candidate explanatory variables are often estimated using a training set of data from closely observed locations but are then applied using covariate data held in a GIS to pbkp_redict the response variable at locations throughout a region. When the regression assumptions hold and the GIS data are free from error, this procedure gives unbiased estimates of the response variable and minimizes the pbkp_rediction mean squared error. However, when the explanatory variables in the GIS are recorded with substantially greater errors than were present in the training set, this procedure does not minimize the pbkp_rediction mean squared error. A theoretical argument leads to the proposal of an adaptation for regression equations to minimize the pbkp_rediction mean squared error. The effectiveness of this adaptation is demonstrated by a simulation study and by its application to an equation for tree growth rate. © 1997 Taylor & Francis Group, LLC.
Año de publicación:
1997
Keywords:
Fuente:
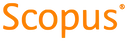
Tipo de documento:
Article
Estado:
Acceso restringido
Áreas de conocimiento:
- Optimización matemática
- Optimización matemática
Áreas temáticas:
- Programación informática, programas, datos, seguridad
- Tecnología (Ciencias aplicadas)
- Geografía y viajes