Adaptive Performance Analysis in IoT Platforms
Abstract:
In this paper, we consider the problem of identifying multiple bottlenecks (a.k.a bottleneck analysis) in IoT Service Platforms. For QoS-constrained applications, IoT Platforms have grown in complexity with non-stationary workloads and inter-task dependencies created by data flows crossing the platform's nodes. These factors create multiple simultaneous 'bottlenecks' (a bottleneck expresses overload in terms of request processing time on a given node, and contributes to QoS degradation). Multi-bottlenecks are non-trivial to analyze since they may escape typical assumptions made in classic performance analysis, such as analysis based on queuing theory models. Solving this analysis problem requires real-time collection and analysis of data that can be massive, and as a result, induce negative impacts on the performance of the NFV-based IoT Platform (NIP) (e.g., use of bandwidth, computing resource, and storage resource). Therefore, it needs to be adapted to the strict minimum allowing effective analysis. We build an adaptive performance analysis method that optimizes bottlenecks' identification for a monitoring overhead budget associated with the different available metrics. Instead of systematically collecting all the NIP metrics, the proposed process determines the best subset of metrics to consider for the efficiency of the performance analysis. The conducted experiments on a practical use case show that the proposed method exhibited high performances of the bottleneck analysis process, in the presence of different bottleneck types and durations, with very few false positives and false negatives.
Año de publicación:
2022
Keywords:
- Machine Learning (ML)
- internet of things (IoT)
- network functions virtualization (NFV)
- performance bottleneck analysis
- Fault localization
Fuente:
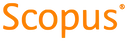
Tipo de documento:
Article
Estado:
Acceso restringido
Áreas de conocimiento:
- Internet de las cosas
- Ciencias de la computación
Áreas temáticas:
- Ciencias de la computación
- Métodos informáticos especiales
- Programación informática, programas, datos, seguridad