Adaptive ε-Ranking on many-objective problems
Abstract:
This work proposes Adaptive ε-Ranking to enhance Pareto based selection, aiming to develop effective many-objective evolutionary optimization algorithms. ε-Ranking fine grains ranking of solutions after they have been ranked by Pareto dominance, using a randomized sampling procedure combined with ε-dominance to favor a good distribution of the samples. In the proposed method, sampled solutions keep their initial rank and solutions located within the virtually expanded ε-dominance regions of the sampled solutions are demoted to an inferior rank. The parameter ε that determines the expanded regions of dominance of the sampled solutions is adapted at each generation so that the number of best-ranked solutions is kept close to a desired number that is expressed as a fraction of the population size. We enhance NSGA-II with the proposed method and analyze its performance on MNK-Landscapes, showing that the adaptive method works effectively and that compared to NSGA-II convergence and diversity of solutions can be improved remarkably on MNK-Landscapes with 3 ≤ M ≤ 10 objectives. Also, we compare the performance of Adaptive ε-Ranking with two representative many-objective evolutionary algorithms on DTLZ continuous functions. Results on DTLZ functions with 3 ≤ M ≤ 10 objectives suggest that the three many-objective approaches emphasize different areas of objective space and could be used as complementary strategies to produce a better approximation of the Pareto front. © 2009 Springer-Verlag.
Año de publicación:
2009
Keywords:
- ε-Ranking
- Selection
- many-objective optimization
- Epistasis
- Adaptation
Fuente:
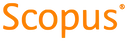
Tipo de documento:
Article
Estado:
Acceso restringido
Áreas de conocimiento:
- Algoritmo
Áreas temáticas:
- Programación informática, programas, datos, seguridad