Adaptive-learning model pbkp_redictive control for complex physiological systems: Automated insulin delivery in diabetes
Abstract:
An adaptive-learning model pbkp_redictive control (AL-MPC) framework is proposed for incorporating disturbance pbkp_rediction, model uncertainty quantification, pattern learning, and recursive subspace identification for use in controlling complex dynamic systems with periodically recurring large random disturbances. The AL-MPC integrates online learning from historical data to pbkp_redict the future evolution of the model output over a specified horizon and proactively mitigate significant disturbances. This goal is accomplished using dynamic regularized latent variable regression (DrLVR) approach to quantify disturbances from the past data and forecast their future progression time series. An enveloped path for the future behavior of the model output is extracted to further enhance the robustness of the closed-loop system. The controller set-point, penalty weights of the objective function, and constraints criteria can be modified in advance for the expected periods of the disturbance effects. The proposed AL-MPC is used to regulate glucose concentration in people with Type 1 diabetes by an automated insulin delivery system. Simulation results demonstrate the effectiveness of the proposed technique by improving the performance indices of the closed-loop system. The MPC algorithm integrated with DrLVR disturbance pbkp_redictor has compared to MPC reinforced with dynamic principal component analysis linked with K-nearest neighbors and hyper-spherical clustering (k-means) technique. The simulation results illustrate that the AL-MPC can regulate the glucose concentrations of people with Type 1 diabetes to stay in the desired range (70–180) mg/dL 84.4% of the time without causing any hypoglycemia and hyperglycemia events.
Año de publicación:
2020
Keywords:
- Latent variable regression
- Adaptive learning
- Insulin delivery system
- Model Pbkp_redictive Control
- Dynamic principal component analysis
Fuente:
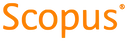
Tipo de documento:
Article
Estado:
Acceso restringido
Áreas de conocimiento:
- Diabetes
- Inteligencia artificial
- Sistema de control
Áreas temáticas de Dewey:
- Fisiología humana
- Enfermedades
- Medicina y salud