Adjustable Access Control Mechanism in Cellular MTC Networks: A Double Q-Learning Approach
Abstract:
The potential of a broad set of applications that might impact different economic sectors rely on their ability to capture, process, and analyze large amounts of data accurately. Concerning wireless networks, one of the main issues that arise in this type of scenarios is access control. In Machine Type Communication scenarios, a vast number of devices could access the network simultaneously, which can harness other types of users. A well-known congestion control method used in cellular networks is access class barring (ACB); it has been designed to allow a large number of users to access the network even under high traffic conditions by delaying their access attempts. However, its performance has to adapt dynamically to traffic conditions while at the same time, providing enough flexibility to administrators to prioritize crucial key performance indicators. We propose a Double Q-learning-based ACB mechanism that adapts dynamically to different traffic conditions when machine-to-machine and human-to-human communications coexist, with three different configurations that allow to adjust the trade-off between successful access probability and mean access delay. The results show that each configuration can reach different levels of QoS related to successful access probability, mean access delay, and mean number of preamble transmissions.
Año de publicación:
2019
Keywords:
- internet of things
- Access Control
- Access Class Barring
- double Q-learning
- 5G
- machine type communications
Fuente:
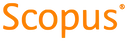
Tipo de documento:
Conference Object
Estado:
Acceso restringido
Áreas de conocimiento:
- Inteligencia artificial
- Ciencias de la computación
- Ciencias de la computación
Áreas temáticas:
- Ciencias de la computación