Distributed optimization of solar micro-grid using multi agent reinforcement learning
Abstract:
In the distributed optimization of micro-grid, we consider grid connected solar micro-grid system which contains a local consumer, a solar photovoltaic system and a battery. The consumer as an agent continuously interacts with the environment and learns to take optimal actions. Each agent uses a model-free reinforcement learning algorithm, namely Q Learning, to optimize the battery scheduling in dynamic environment of load and available solar power. Multiple agents sense the states of the environment components and make collective decisions about how to respond to randomness in load, intermittent solar power using a Multi-Agent Reinforcement Learning algorithm, called Coordinated Q Learning (CQL). The goals of each agent are to increase the utility of the battery and solar power in order to achieve the long term objective of reducing the power consumption from grid.
Año de publicación:
2015
Keywords:
- Battery scheduling
- Optimization
- CQ-learning
- Solar micro-grid
- Multi-agent reinforcement learning
Fuente:
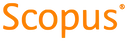
Tipo de documento:
Conference Object
Estado:
Acceso abierto
Áreas de conocimiento:
- Energía renovable
- Aprendizaje automático
- Energía renovable
Áreas temáticas:
- Física aplicada
- Métodos informáticos especiales