Droplet evolution pbkp_rediction in material jetting via tensor time series analysis
Abstract:
Material Jetting (MJ) process is an additive manufacturing process that is able to produce structures with high resolutions. The performance and quality of the MJ printed parts extensively rely on the droplet morphology and behavior. However, obtaining consistent and stable droplet morphology and behavior is difficult because the droplets are very sensitive to different material and process parameters. Testing/studying all these parameter combinations is time-consuming due to the high-experimental and high-computational costs. We thus study the pbkp_rediction of droplet behaviors under different material and process parameters. To achieve this, we propose to leverage the underlying relationships shared across droplet evolution behaviors with diverse material and process parameters (referred to as “cross-linked” relationship hereafter) as well as the spatial–temporal relationships of droplet evolution, and capture these with the Network of Tensor Time Series (NET3). The distinct droplet behaviors are regarded as co-evolving time series (i.e., Tensor Time Series (TTS)) since they share the same physics principles. In particular, we capture the cross-linked and spatial relationships of TTS by the Tensor Graph Convolutional Network (TGCN), and capture the temporal relationship of TTS by the Tensor Recurrent Neural Network (TRNN), respectively. The features from TGCN and TRNN are passed to Multilayer Perceptron (MLP) for pbkp_redicting future droplet behaviors. The proposed methodology is demonstrated with simulated (i.e., physics-based models) and experimental (i.e., MJ printing observation with vision system) droplet evolution videos and is able to accurately and efficiently make pbkp_redictions for seen (i.e., forecasting for a future time) and unseen (i.e., new droplet evolution sequence pbkp_rediction) material/process parameters.
Año de publicación:
2023
Keywords:
- Droplet evolution
- deep learning
- Material jetting
- additive manufacturing
- Tensor time series
Fuente:
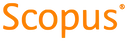
Tipo de documento:
Article
Estado:
Acceso restringido
Áreas de conocimiento:
- Optimización matemática
- Ciencia de materiales
Áreas temáticas:
- Ciencias de la computación