Dynamic control of the number of crossed genes in evolutionary many-objective optimization
Abstract:
When multi-objective evolutionary algorithms (MOEAs) are applied to many-objective optimization problems (MaOPs), genetic diversity of solutions in the population significantly increases to explore the true Pareto optimal solutions distributed in broad region of variable space. In MOEAs, if genetic diversity of solutions in the population become noticeably diverse, conventional crossovers become too disruptive and decrease its effectiveness. To realize effective genetic operation in MaOPs, crossover controlling the number of crossed genes (CCG) has been proposed. CCG controls the number of crossed genes by using an user-defined parameter α. CCG using a small α remarkably improves the search performance of MOEA especially in MaOPs by restricting the number of crossed genes. The conventional CCG uses a fixed value of α throughout a single run of MOEA, so that the number of crossed genes does not change during the solutions search. However, since the population dynamically changes during the evolution, the optimal number of crossed genes will change during the solutions search. To further improve the search performance of MOEAs in MaOPs, in this work we propose a dynamic CCG which dynamically controls α according to the number of generations. Simulation results focusing on many-objective 0/1 knapsack problems show that dynamic CCG reducing α during the solutions search achieves higher search performance than the conventional CCG using an optimal fixed α. Also, we show that convergence and diversity property of the obtained solutions are emphasized by dynamic control of α. © 2012 IEEE.
Año de publicación:
2012
Keywords:
Fuente:
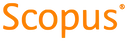
Tipo de documento:
Conference Object
Estado:
Acceso restringido
Áreas de conocimiento:
- Algoritmo
- Evolución
- Algoritmo
Áreas temáticas:
- Programación informática, programas, datos, seguridad
- Relaciones internacionales
- Biología